
Remember that covariance is applied to 2 assets. Just keep the focus on the article and everything will be ok!įirst, you need to understand what covariance and correlation are. You then started to see a lot of use of covariances and correlations, but strangely enough, you see those two words with the prefix " auto" and you get frightened!ĭon’t worry, this article will help you understand their details. You might have encountered yourself trying to learn the Autoregressive Moving Average (ARMA) model.
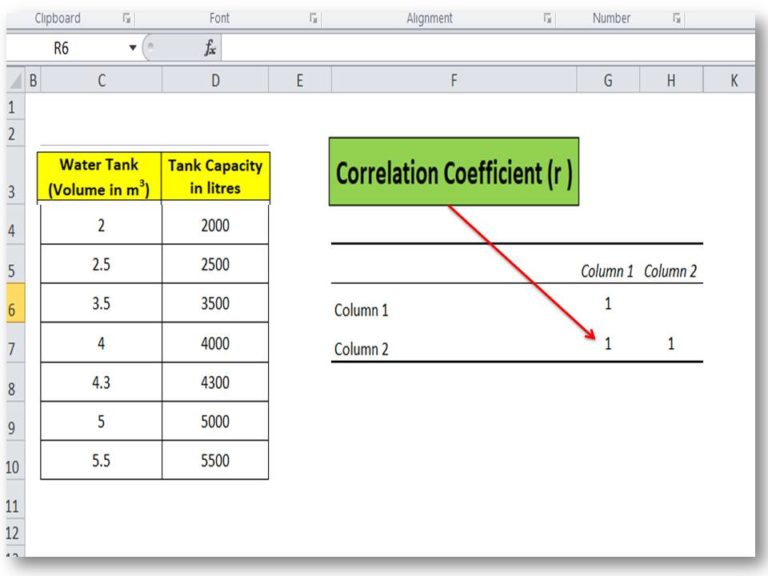
394y i-1 + ε i where σ 2 = 1.421703.įigure 1 – Estimation of AR(1) coefficientsĪs we can see, the process coefficients are pretty close to the original coefficients used to generate the data in column B ( φ 0 = 5, φ 0 =. This estimate of the time series is the process y i = 4.983 +. From these, we can estimate the process coefficients as shown in cells G8:G10. We next calculate the mean, variance and PACF(1) values.

The first 8 of 100 data elements are shown in column B of Figure 1. Alternatively, we use the values μ, γ 0, π 1…, π p (PACF values), which it turns out are equivalent.Įxample 1: Use the statistics described above to find the coefficients of the AR(1) process based on the data in Example 1 of Autoregressive Processes Basic Concepts. One approach is to use the Yule-Walker in reverse to calculate the φ 0, φ 1, …, φ p, σ 2 coefficients based on the values of μ, γ 0, …, γ p (ACF values). We illustrate the first of these approaches on this webpage. We now show how to calculate the process coefficients using the following techniques: (1) estimates based on ACF or PACF values, (2) using linear regression and (3) using Solver. Suppose that we believe that an AR( p) process is a fit for some time series.
